Statistical data analysis
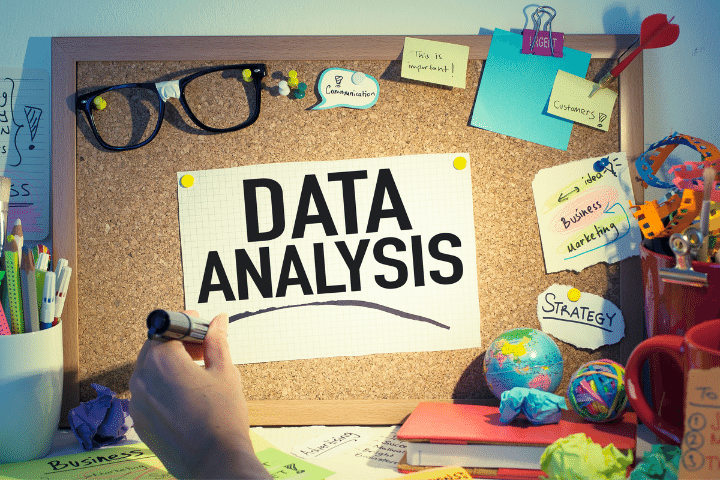
Data and information is undoubtedly one of the most important resources for any project or company. Thanks to the compilation and subsequent analysis we can make decisions that optimize our project and make it grow.
But... What exactly is data analysis and how is it carried out? In this article we tell you everything you need to know so that you can get an idea of how data and information are analyzed.
What is data analysis?
Data analysis is defined as a process of cleaning, transforming and modeling data to discover useful information for business decision making. The purpose of data analysis is to extract useful information from the data and make the decision based on the data analysis.
A simple example of data analysis is when we make any decision in our daily lives, thinking about what happened last time or what will happen when choosing that specific decision. This is nothing more than analyzing our past or our future and making decisions based on it. To do this, we collect the memories of our past or the dreams of our future. This is nothing more than data analysis. The same thing that an analyst does for business purposes is called data analysis.
In this tutorial, you will learn:
- Why data analysis?
- Data analysis tools
- Types of data analysis: Techniques and methods
- Data analysis process
Why data analysis?
To grow your business, even to grow your life, sometimes all you need is Analysis!
If your business is not growing, then you have to look back and recognize your mistakes and make a plan again without repeating those mistakes. And even if your business is growing, then you have to look ahead to make the business grow more. All you have to do is analyze your business data and business processes.
Data analysis tools
Data analysis tools make it easier for users to process and manipulate data, analyze relationships and correlations between data sets, and also help identify patterns and trends for interpretation. Here is a complete list of tools used for data analysis in research.
Types of data analysis: Techniques and methods
There are several types of Data Analysis techniques depending on the business and technology. However, the main Data Analysis methods are:
- Text analysis
- Statistic analysis
- Diagnostic analysis
- Predictive analysis
- Prescriptive analysis
Text analysis
Text analysis is also known as data mining. It is one of the data analysis methods to discover a pattern in large data sets using databases or data mining tools. It is used to transform raw data into business information. There are Business Intelligence tools on the market that are used to make strategic business decisions. Overall, it offers a way to extract and examine the data and derive patterns and eventually interpretation of the data.
Statistic analysis
Statistical Analysis shows "What's wrong?" using past data in the form of dashboards. Statistical Analysis includes the collection, analysis, interpretation, presentation and modeling of data. Analyzes a set of data or a sample of data. There are two categories of this type of analysis: descriptive analysis and inferential analysis.
Descriptive analysis
analyzes complete data or a sample of summarized numerical data. Displays the mean and deviation for continuous data, while the percentage and frequency for categorical data.
Inferential analysis
analyzes complete data samples. In this type of analysis, different conclusions can be found from the same data by selecting different samples.
Diagnostic analysis
Diagnostic Analysis shows "Why did it happen?" finding the cause from the information found in the Statistical Analysis. This Analysis is useful to identify patterns of data behavior. If a new problem comes to your business process, then you can search this Analysis to find similar patterns of that problem. And you may have possibilities to use similar recipes for new problems.
Predictive analysis
Predictive analytics shows “what is likely to happen” using past data. The simplest example of data analysis is that if last year I bought two dresses based on my savings and if this year my salary increases twice as much, then I can buy four dresses. But, of course, it is not that easy because you have to think about other circumstances, such as the possibility that clothing prices will increase this year or that, instead of dresses, you want to buy a new bicycle, or that you need to buy a house .
In this case, Analysis makes predictions about future results based on current or past data. The forecast is just an estimate. Its accuracy is based on how much detailed information you have and how deep you go into it.
Prescriptive analysis
Prescriptive Analytics combines insight from all previous Analytics to determine what action to take on a current problem or decision. Most data-driven companies use Prescriptive Analytics because predictive and descriptive Analytics are not enough to improve data performance. Based on current situations and problems, they analyze data and make decisions.
Data analysis process
The process of data analysis is nothing more than collecting information by using a suitable application or tool that allows you to explore the data and find a pattern in it. From this information and data, decisions can be made or definitive conclusions can be obtained.
Data analysis consists of the following phases:
- Data Collect
- Data Cleaning
- Analysis of data
- Data interpretation
- Data visualization
Data Collect
First of all, you have to think about why you want to do this data analysis. All you need is to find out the purpose or objective of doing data analysis. You have to decide what type of data analysis you want to do. In this phase, you have to decide what to analyze and how to measure it, you have to understand why you are investigating and what measures you have to use to do this Analysis.
Data Collect
After requirements gathering, you will have a clear idea of what things you have to measure and what your conclusions should be. Now is the time to collect the data based on the requirements. Once you collect data, remember that the data collected must be processed or organized for analysis. As you collect data from various sources, you should keep a record of the date of collection and the source of the data.
Data Cleaning
Now, the data collected may not be useful or may be irrelevant to your analysis objective, so it must be cleaned. The data collected may contain duplicate records, blank spaces or errors. The data must be clean and error-free. This phase must be carried out before the analysis, since, thanks to the cleaning of the data, the result of the analysis will be closer to the expected result.
Analysis of data
Once the data is collected, cleaned and processed, it is ready for analysis. By manipulating the data, you may discover that you have the exact information you need, or that you need to collect more data. During this phase, you can use data analysis tools and software to help you understand, interpret, and draw conclusions based on the requirements.
Data interpretation
After analyzing the data, it is time to interpret the results. You can choose how to express or communicate your data analysis, whether simply with words or perhaps with a table or graph. Next, use the results of your data analysis process to decide your best course of action.
Data visualization
Data visualization is very common in everyday life; They often appear in the form of tables and graphs. In other words, the data is displayed graphically so that the human brain can understand and process it more easily. Data visualization is often used to uncover unknown facts and trends. By observing relationships and comparing data sets, you can find a way to find out meaningful information.
Summary:
- Data analysis is a process of cleaning, transforming and modeling data to discover useful information for business decision making
- Types of data analysis are textual, statistical, diagnostic, predictive and prescriptive analysis
- Data analysis consists of data requirements gathering, data collection, data cleaning, data analysis, data interpretation and data visualization.